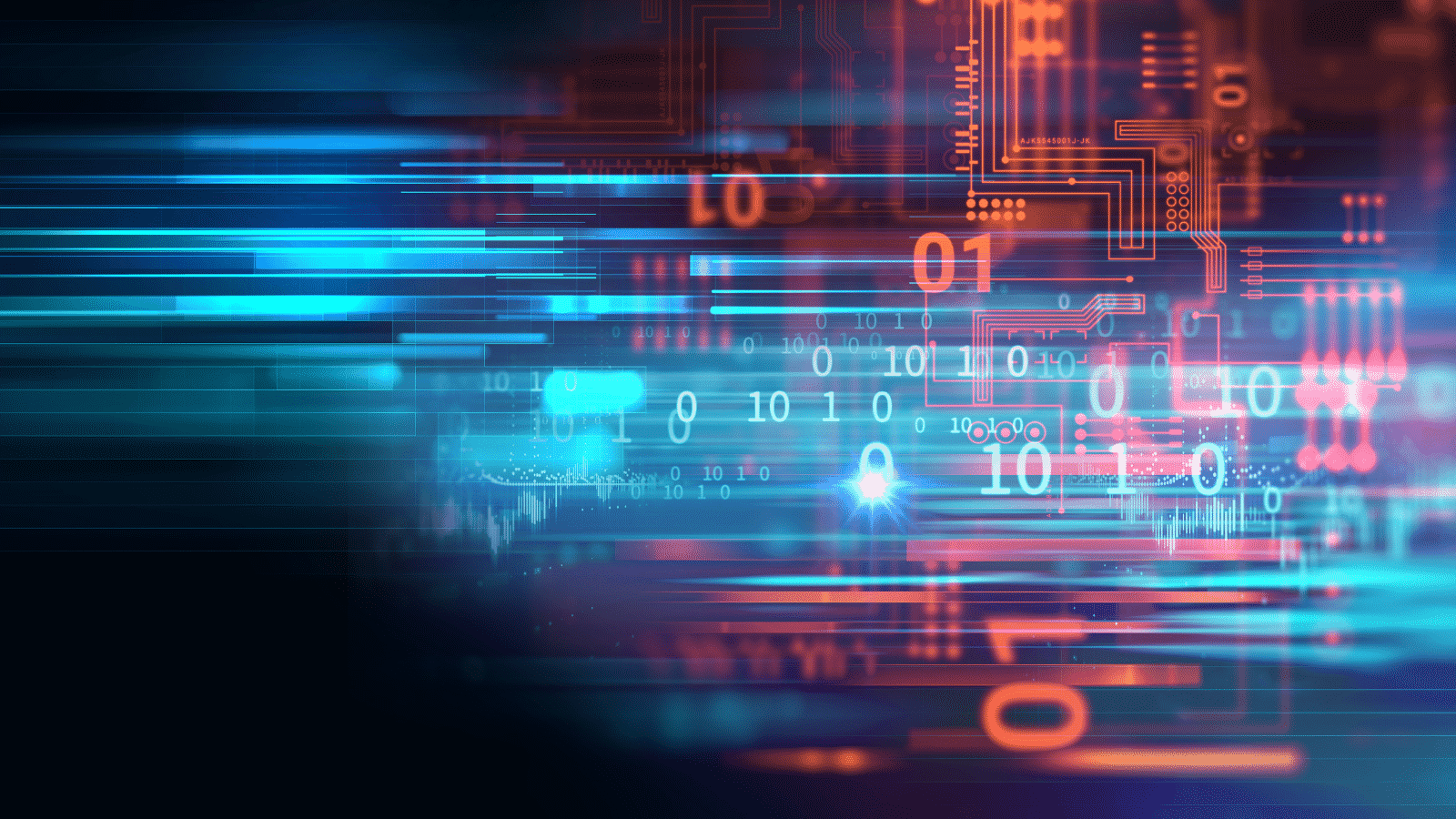
Data Cleansing & Data Conversion: A Primer
Data Cleansing (Cleansing) and Data Conversion (Conversion) are without a doubt intertwined disciplines. The sections which follow provide clarity regarding terminology used in each of these separate fields. Additionally, the discussion explores the combined importance of each to enterprise-wide Data Quality Management (DQM).
The critical need for comprehensive cleansing is simple: the requirement to address dirty or inaccurate enterprise data. Low quality data is estimated to cost business organizations up to 12% of revenue per year according to Forbes. In its 2019 Global Data Management research report, Experian noted that ninety-five percent of organizations see impacts in their organizations from poor-quality data. The end goal of cleansing processes: data hygiene.
Cleansing is a prerequisite to conversion to the extent that legacy data does not match desired specifications. Data is most often converted in order to migrate the data from one system (the source) to another (the target). Frequently data being extracted from the source contains a variety of inconsistencies and errors that must be addressed via cleansing before it is moved to the target.
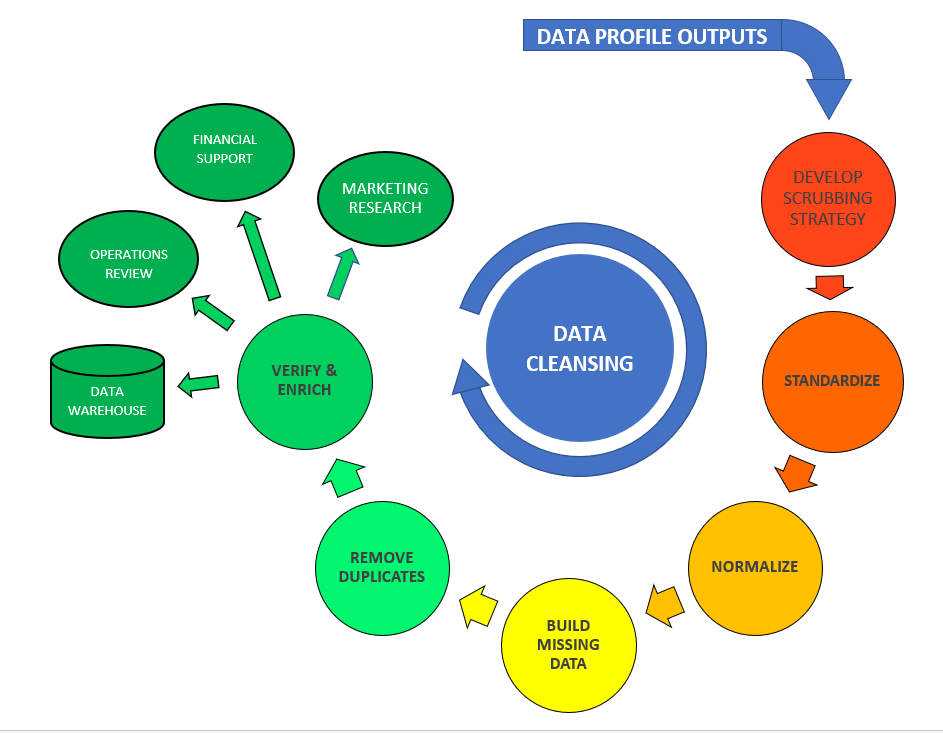
Cleansing
Respecting the adage garbage in, garbage out, executive management are reticent to use inaccurate data in an attempt to develop information necessary to formulate sound strategic decisions. In short, poor quality prevents data from being the strategic asset it should necessarily be. Absent application of comprehensive cleansing processes, underlying data of subpar quality will result in wasted resources and incurrence of additional unnecessary costs.
Cleansing is the foundational component of Data Quality Management (DQM). Properly engineered and structured, strategies are developed and employed to correct data deficiencies whether due to:
- human error
- disparate data sources
- lack of adequate technology
- lack of inter-departmental data sharing
As discussed above, cleansing is a necessary prerequisite to conversion; as well, it is a critical component of a migration effort in some cases. It is also a fundamental component of a data enrichment process whereby data from multiple sources is aggregated in order that it can be used in a number of ways across the enterprise. Aggregation of data from multiple sources increases the risk that inconsistencies will be introduced which will decrease the validity – and thus the inherent value – of the resulting dataset.
It follows that cleansing is a crucial component in building and maintaining a data pipeline; it provides the means to address inconsistencies whether the pipeline be used to establish and continually replenish a data warehouse or for another purpose.
Cleansing consists of two separate sub-disciplines: Data Profiling (Profiling) and Data Scrubbing (Scrubbing).
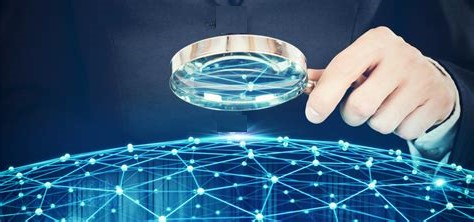
Profiling. Assessing the character of existing data is the first step to improving underlying quality as well as providing additional benefits to an organization outlined below. It is vital to determining data quality – the degree to which it conforms to standards or patterns of an entity’s data governance policies and procedures.
Profiling processes are executed to determine the existence of errors or anomalies in data sets which decrease validity and lessen its overall value. As a first step, Profiling requires a comprehensive review of datasets and of data table schema to derive business rules inherent therein.
As noted, data quality is diminished by common errors. Anomalies may include: errors conforming to data type, missing values, excess length of values, the absence of discrete values, and values outside of a specified valid range; also discovered may be data that is not unique or does not meet abstract type criteria.
Particulars of errors and anomalies discovered in existing data will provide crucial intelligence necessary to tailor methods and essential algorithms to ensure that errors are identified and corrected on a continuous basis - at the source . This is the case whether the source be user entry or an ETL (Extract, Transform, Load) pipeline.
In addition to determining the presence of errors or anomalies in a dataset, profiling permits calculation of attribute specific statistics to lay the foundation for further analysis by marketing and/or financial analysts. Determination of the extent to which data can be exploited for additional purposes than currently utilized increases the return on investment of any data profiling initiative.
Profiling also enables collection of informative summaries of unique characteristics which in turn permit discovery of business knowledge embedded in the data. Additional benefits of data profiling include:
- Understanding the risk and issues involved with integrating data into new applications
- Determining data challenges early in a data intensive project thereby diminishing the risk of project delays and cost overruns
- Ascertaining functional dependencies amongst data attributes through cross-column analysis
- Identifying foreign-key candidates, through inter-table analysis, necessary to normalize data for storage stored in a relational database
- Discovering metadata regarding the source database
- Assessing whether known metadata accurately describes the actual values in the source database
- Providing an enterprise view of all data critical to data management
- Determining the value-added of external candidate data sources under consideration to enrich existing data as regards both quality and granularity
As noted, Profiling results provide an in-depth understanding of underlying data necessary to formulate strategies on which Scrubbing processes will be tailored. Doing so, it lays the foundation for development of actionable strategies to take control of data quality management in the digital age.
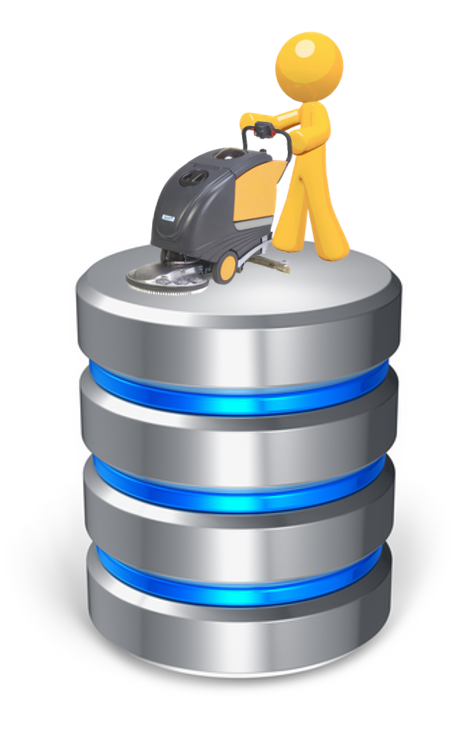
Scrubbing. Scrubbing processes are designed and used to cleanup dirty data based on knowledge derived through Profiling. In short, Scrubbing is the process of fixing corrupted, incorrectly formatted, or incomplete data within a dataset. It extends to correcting issues that may prevent standardizing the data into a single format or that may distort analysis performed on the dataset.
More specifically, strategies on which Scrubbing processes are tailored are formulated from intelligence gathered through successful Profiling; this includes an in-depth knowledge of business rules inherent in the schema of each target table.
Scrubbing requires data to first be loaded into staging tables. Errors and inconsistencies are addressed through processes tailored to:
- validate and transform data into the correct data type; for example, to validate dates or binary data held in text fields prior to loading into a date-time type or boolean type field respectively
- determine that non-foreign key data fails within acceptable data ranges
- validate codes to be used as foreign keys; incorrect codes will prevent use of key constraints on which relationships are to be based. For example, when used in a sales order system as a foreign key in the CustomerOrders table, each CustomerID must have a matching entry in the Customers primary table.
- format data to a common form as per conversion specifications; examples may include the addition, or removal, of spaces in tax numbers or phone numbers
- fill in missing data; for example, a deceased pension fund participant’s data of death might be populated by reference to data provided by the Social Security Administration
- correct typographical errors
- remove duplicates
- determine and correct field level data which is contradictory or mutually exclusive; for example, city and state values which conflict with the corresponding zip code
Some categories of information require implementation of more than one of the actions listed above to fulfill a Scrubbing initiative. Hygiene and standardization of address data, for example, may require validation of codes, transformation to a standard format, completion of missing data, correction of typographical errors, removal of duplicates, and resolution of conflicting data.
In summary, analysis of an organization’s data assets performed during cleansing is key to the designing data quality control functionality built into each IT component necessary to detect and correct errors at the source; the efficacy of such “front-end” edits ensures the quality of the entity’s data on an ongoing basis. This remains the case whether the data is brought in directly through a data portal (e.g., an online sale) or is gleamed from external data source through an ETL pipeline. Cleansing is a foundational prerequisite to building fact and dimension tables of a data warehouse as well as to cleaning up dysfunctional data housed in an existing data warehouse.
It is vitally important that project teams tasked with developing internal IT systems – whether creating a new system, enhancing existing functionality, or replacing legacy programs – have individuals with expertise in Profiling and Scrubbing onboard at the onset of the Systems Analysis project phase.
Procedures employed to Profile and to Scrub data are critical to increasing the quality of data generally; properly executed, these procedures unlock the value of information resources flowing into, as well as internal to, an organization.
Understanding key aspects of these related areas highlights the importance of each to an entity; as well, it provides background to better understanding the positive results Synergy has achieved in these areas critical to Data Quality Management.
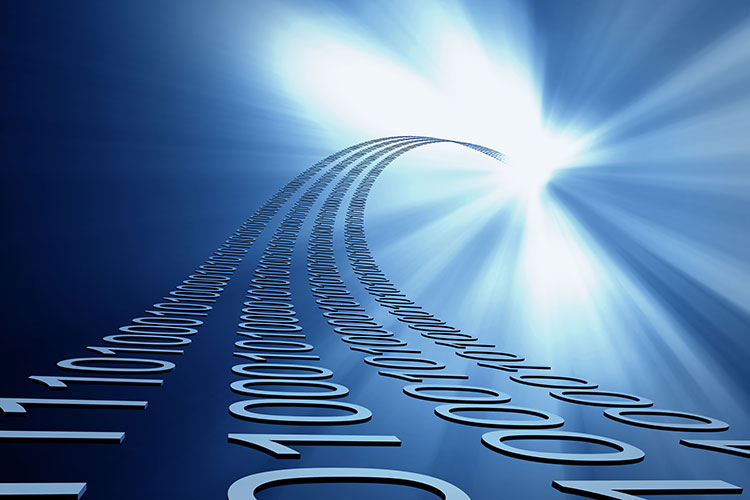
Conversion
Data conversion is performed in order to migrate the data from one system (the source) to another (the target). By definition clean data, often a result of carefully crafted cleansing processes, is a necessary component of conversion.
Conversion involves extracting clean data from a legacy source, transforming it based on tightly tailored specifications, and loading the transformed data into a target system. Simply, it is the transformation of data from one format to another.
Typically, the purpose of a conversion project is to move data from a legacy system to a newly developed, or purchased, replacement system. The new system, though often significantly enhanced, performs an underlying function similar in nature to its predecessor.
A conversion project might also result from an initiative to integrate data from across the enterprise in order to permit its use in a different or more valuable manner. For example, an entity might seek to merge data from financial, sales, and operations systems to create a single customer view to facilitate its marketing efforts.
Data to be converted must be clean in order to conform to constraints setup to enable ongoing data quality management. Such constraints include those to
- prevent nulls or empty strings
- ensure values are unique (while permitting null or empty values)
- provide for a primary key that is both unique and not null
- permit enforcement of referential integrity through the use of foreign and primary keys
- provide data range checks; for example, preventing a “date of application” from being input having a value greater than the date on which it is entered
Subsequent to cleansing, data held in temporary staging tables is converted and subsequently moved into a development database with tables mirroring those to which it will be migrated. Mapping tables are required to convert certain data. Conversion of a legacy four-digit CustomerID to a six-digit code, for example, will require reference to a mapping table to facilitate conversion of the CustomerOrders table.
The complexity of a conversion project can be greatly influenced by the quality of the legacy data. If the legacy data has not previously been cleansed, a conservative approach to conversion requires basic Profiling processes to be employed to determine if Scrubbing is necessary and cost effective.
The final step of any conversion process is the transfer of data from the development staging tables into which converted data has been written to the production database. It is imperative that migration processes ensure all records are accounted for and that numeric totals are verified whether using automated or manual auditing processes.
Synergy’s capability and competence in the fields of Cleansing and Conversion is detailed below in the section headed Synergy's Expertise In The Fields Of Data Cleansing & Conversion.